I’ve worked with companies that obsessed over their Glassdoor reviews like Daniel Day-Lewis obsesses over every aspect of his character. I’ve also worked at companies that couldn’t care less and saw the platform as a way for disgruntled employees to take parting shots when things didn’t work out.
Whether your brand is on one side of the spectrum or more in the middle, you need to start paying attention to the roles, content and context of your inbound reviews and ratings because as you’ll see in this case study, it can have global implications.
Ranking Global IT Firms
Having come from 2.5 great years at TCS, I decided to test RankBee’s (in development) AI brand intelligence tool for the top global IT firms. Similar to the rating scale in my post on Luxury Brands, I pulled in global brand metrics and then segmented the results for a competitive set of Accenture, TCS, Infosys, Cognizant and Deloitte.
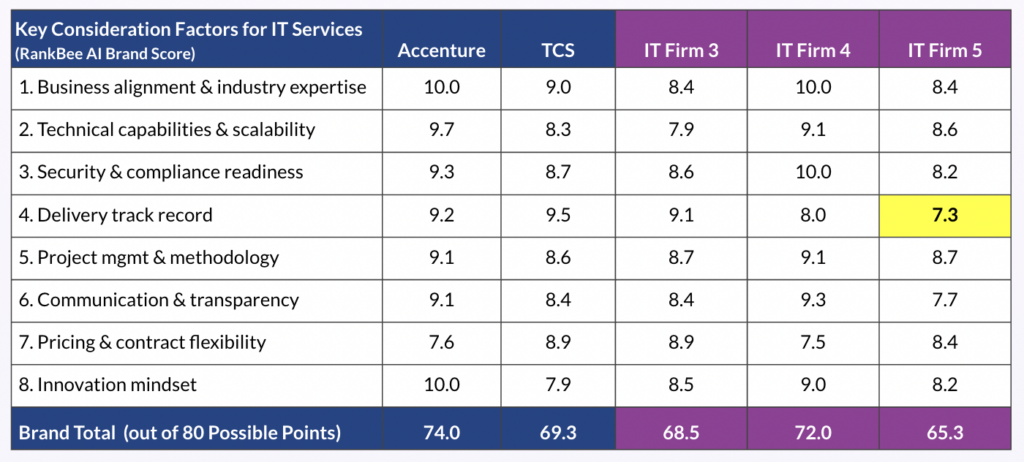
After putting a myriad of data into our system, the numbers showed Accenture was the overall category leader and my old firm TCS was 3rd in the group based on scores. Most of the brand factor scores were between 8.5-9.5 but what stuck out was that the lowest score for any of these factor metrics was on delivery which is the lifeblood of IT client servicing.
You’ll notice the number is under the heading ‘IT Firm 5’ because selfishly I want to have some level of mystery/engagement as to what the brand could be and also just putting it there, I feel like some people would read it and say “Makes sense” or “I’ve heard that about them” without diving deeper into why this is being generated by LLMs in the first place.
“Inconsistent Delivery and Slower to Innovate”
I began diving into the reason for the low score and first looked at the sources being pulled in to generate both the positive and negative sides of the score/perception.
On the positive side, external sources like press releases, C level interviews and industry awards for client satisfaction were being pulled in – alongside the client’s own case studies and investor relations pages talking about internal client survey results.
For most brands, this would form a very positive score of at least 8.5 but unfortunately for this IT firm, there was quite a bit of content that subtracted from the score – bringing it nearly a full point below their next competitor.
Glassdoor and Indeed’s Impact of AI Brand Perception
When looking at what was forming the basis for the low delivery score, there were a multitude of citations for Glassdoor and Indeed and when you think about it, it really makes sense as to why these sites would have the outsized impact they have.
The cited sources were review category pages for roles like delivery manager, project manager, delivery lead, account manager etc… and as I read through page after page of detailed, largely critical employee experiences, it really hit me as to why LLMs would think these are a rich data source for informing their opinion on delivery.
First you have the context that the material is coming from someone whose role would have been intimately familiar with that particular attribute of the consideration process – in this case project delivery and management.
Second is that reviews, especially on the employment side, tend to highlight specific, contextually relevant items in a long form fashion that would give LLMs even further contextual elaboration as to the company’s ability to meet certain functions.
Finally a single review won’t be enough to establish a trend but with the number of employees these firms have spread across multiple geographies and the levels of turnover natural to these operations, there’s a considerable volume of data and with that potentially enough material for LLMs to start seeing trends.
Could the Score Actually Impact Deal Flow?
IT services at this level are largely sold through relationships where you’ll have 10-15 people on an account constantly meeting and interacting with clients and those connections are largely what drive deal flow vs an enterprise brand going to Google or ChatGPT and trying to find service providers.
However as companies continue to look for ways to further reduce overhead, procurement functions are likely to be more and more AI driven and it’s conceivable that in the future, software will automatically determine which approved vendors can and cannot bid for a contract.
In that reality, which I feel is not too far away, companies like this one would see their lower delivery score begin to impact their sales pipeline in meaningful ways and may not even realize why until it’s too late.
So what should brands do?
Brands need to pay closer attention to macro trends that emerge from review sites and give them proper consideration rather than ignoring or getting into the habit of looking at a site like Glassdoor or Yelp as a place for venting frustration.
As seen in this example, a trend established over time with contextually relevant content from contextually relevant individuals can have an outsized impact on a global 100,000 employee firm.
Now that we can see how this impacts LLMs, brands should incorporate public exit “interview” trends as a core part of their annual internal reviews and leverage trends to better inform areas of structural and process improvement going forward to buck trends and establish better baselines.
Keep in mind that LLMs won’t laser focus on a single source to form a perception and in this case, there is a considerable amount of positive content talking about this company’s delivery strength – however brands need to do what they can to impact sources of doubt and continue to build on sources of strength.
Post originally written by me on LinkedIn April 1, 2025.